In the high stakes world of digital multimedia communication, one security method clearly stands out above the rest: image steganography, or the art of hiding data within an image.
Simply put, image steganography involves embedding any form of data, be it text, images or video, within a cover image such that there are no changes to the cover image that are perceptible to the naked eye.
The process is already used in a range of fields including defence, industry, social media, intelligence and medicine. Examples span from the beneficial in items such as smart identity cards, where an individual’s personal details are embedded in their photograph, to the unethical where cyber-criminals can embed viruses, spam or malicious links in these images instead.
A team of scientists at the University of Wollongong have looked at how to make the image steganography process even better, using machine learning to increase the size of the data that can be embedded in an image while making it harder for eavesdroppers to spot the secret data.
The enhanced embedding process was also able to create a secret key used to retrieve the hidden data at a later date, further enhancing security and reducing the chance of hacking by intruders.
Hide and seek against our human vision
Inas Jawad Kadhim, lead author of the study1, told Lab Down Under that steganography in general made it difficult to detect the existence of hidden data through standard analysis methods of the cover image with the embedded data, called the stego-image.
“Due to this reason, steganography can be considered as a better approach than that of cryptography in hiding some information in plain sight,” she said.
In conventional steganography approaches, higher payload capacity (or the amount of data being embedded) can causes distortions in the stego-images themselves. This distortion can then affect the imperceptibility, a measure of how easily the human eye can distinguish between the cover image before and after it has secret data embedded within.
“The data can be embedded over common natural images, and it will be quite tough to detect or retrieve the embedded information unless the communicated image is affected by distortions or embedding artefacts,” Kadhim said.
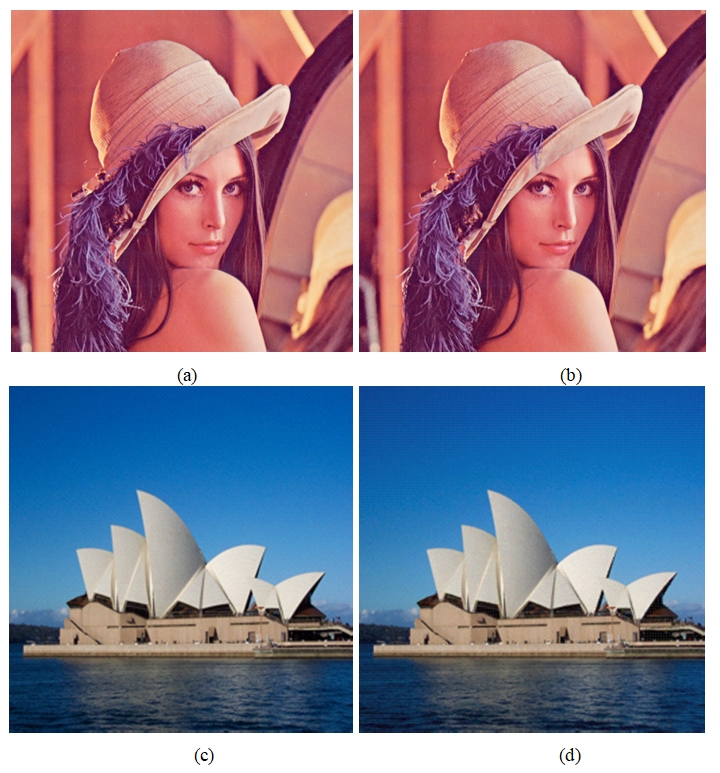
The characteristics of the cover image dictate how easily these distortions can be spotted by individual viewers, with current steganographic techniques aiming at minimising distortions, she added.
“The human visual system is less sensitive detecting changes in textured regions compared to smooth regions. Hence, the majority of state-of-the-art high capacity embedding approaches try to minimise stego-image distortions by focusing on embedding more over the edges and textured regions than over smooth regions.”
However, these approaches had limitations, she added, because the payload capacity depended on the cover image itself. This meant that high payloads caused drops in imperceptibility, which meant the process was ineffective at hiding the image.
Machine learning and the art of online stealth
In creating the enhanced algorithm, Kadhim and her team used a signal processing method known as Dual-Tree Complex Wavelet Transforms (DT-CWT) enhanced with machine learning to select which sections of the cover image were more suited for the image embedding process.
Effectively, the algorithm was shown a vast number of training images, learning which image sections were textured and which were smooth, selecting the former for embedding while discarding the latter.
“The selection of optimal blocks from the cover data by using machine learning helps to maintain a high visual quality even when the embedding requirement is high. Our machine learning-based cover block selection yields much better results rather than conventional steganographic systems,” Kadhim said.
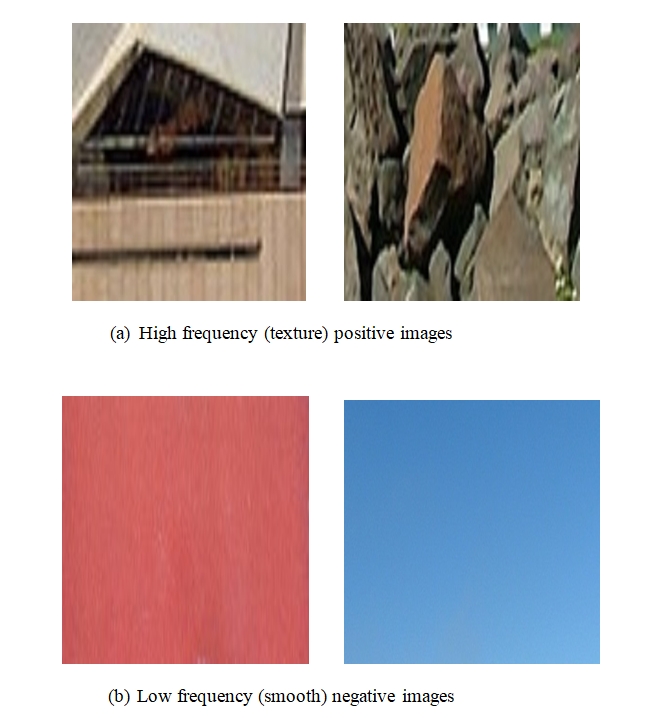
The algorithm was also improved to increase payload capacity and imperceptibility while enhancing security and minimising retrieval errors (effectively any errors in embedded data created when it is extracted from the cover image).
“Different cover regions possess different statistical features and often vary based on the cover image contents. Our machine learning-based cover region selection helps to analyse these features, and select regions which give fewer embedding errors as well as low retrieval errors.”
The algorithm could still be improved through future research, Kadhim said, because DT-CWT itself contained multiple subband planes, some of which may not work efficiently while embedding any secret data.
“Hence, the region selection can be further upgraded to find the suitable subbands from all available cover image subbands using better machine learning approaches.”
Further improvements could also be made by implementing pre-processing techniques that reduced time and memory spent in embedding the secret data, she told Lab Down Under.
“We tried to develop an embedding mechanism which can work over textured as well as smooth regions in the cover media without creating detectable stego-image distortion, and with minimal secret data retrieval error at higher capacity. In such high imperceptivity conditions, an advanced high capacity steganographic system can be further designed which can extract the secret data from the cover image itself.”
Kadhim conducted this research under the supervision of Dr Prashan Premaratne and Dr Peter James Vial.
Author’s note: If you enjoyed this article, you can follow Lab Down Under on Facebook or support me on Patreon. I also have my own personal Twitter account where I’ll be sharing my latest stories and any other items of interest. Finally, you can subscribe here to get my weekly blogs in your inbox.
1 High capacity adaptive image steganography with cover region selection using dual-tree complex wavelet transform, Cognitive Systems Research, Volume 60, May 2020
Featured image: Beyond Resolution by Rosa Menkman used under the 2.0 Generic (CC by 2.0) creative commons licence.